Google DeepMind Unveils SigLIP 2 Vision-Language Model
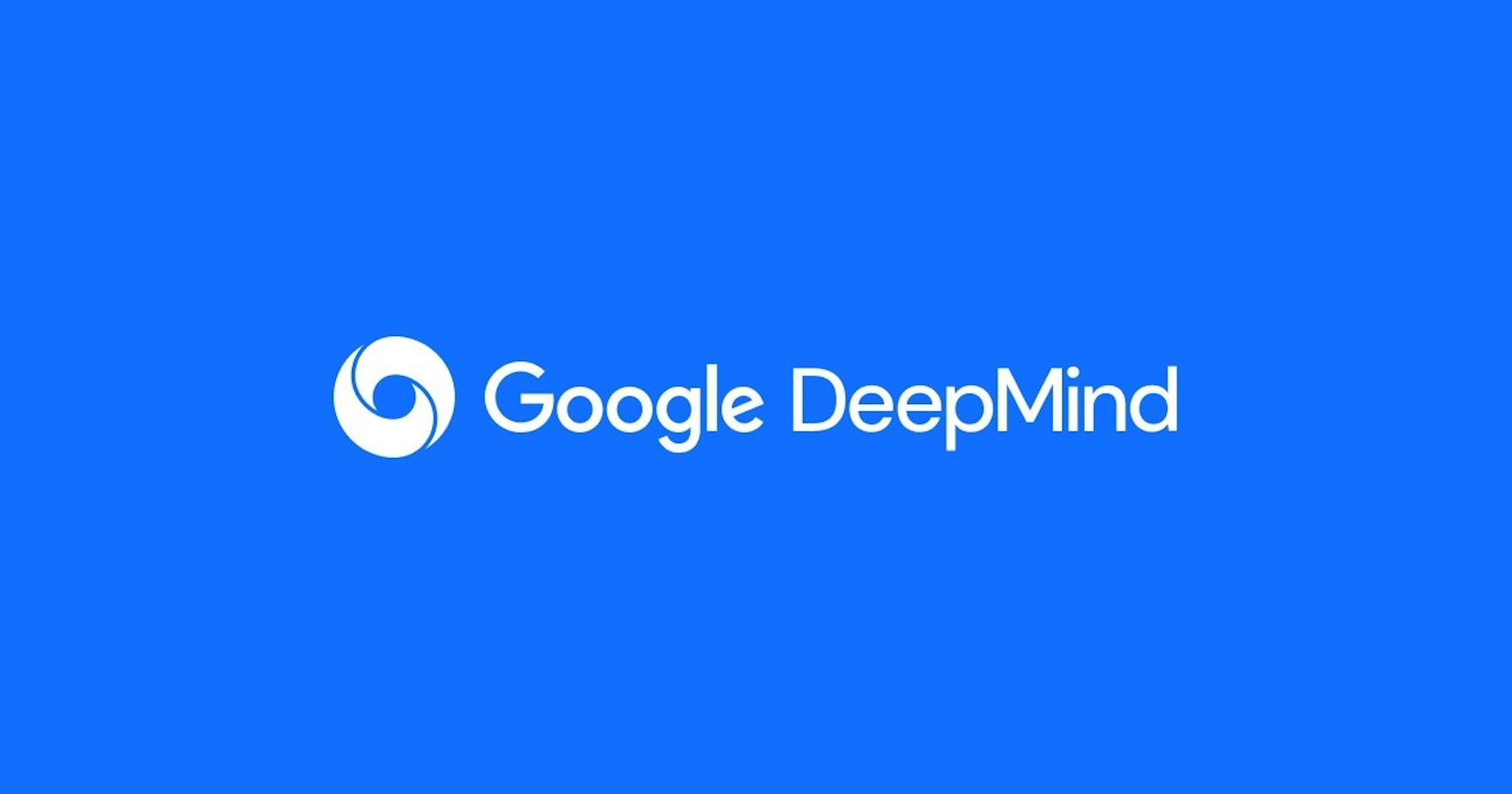
Google DeepMind has released SigLIP 2, a new family of multilingual vision-language encoders designed to enhance semantic understanding and localization, according to MarkTechPost. SigLIP 2 builds on the original image-text training objective by integrating captioning-based pretraining with self-supervised methods like self-distillation and masked prediction.
The model employs a sigmoid loss function, which balances the learning of global and local features, and includes a decoder-based loss for tasks such as image captioning and region-specific localization. This approach improves performance in dense prediction tasks. Additionally, the NaFlex variant supports native aspect ratios, maintaining image integrity across various resolutions.
SigLIP 2 demonstrates consistent improvements over previous models in benchmarks like zero-shot classification and multilingual image-text retrieval tasks. It also shows reduced bias in object-to-gender associations, thanks to de-biasing techniques used during training. The model's ability to handle tasks requiring detailed spatial reasoning and robust text alignment makes it a versatile tool for applications such as OCR and document processing.
The release of SigLIP 2 on Hugging Face simplifies the integration of advanced vision-language capabilities into existing systems, reducing the need for separate models or extensive fine-tuning. This unified approach enhances the model's applicability in real-world scenarios, offering a strong foundation for future vision-language research.
We hope you enjoyed this article.
Consider subscribing to one of our newsletters like Daily AI Brief.
Also, consider following us on social media:
Subscribe to Daily AI Brief
Daily report covering major AI developments and industry news, with both top stories and complete market updates
Market report
2025 Generative AI in Professional Services Report
This report by Thomson Reuters explores the integration and impact of generative AI technologies, such as ChatGPT and Microsoft Copilot, within the professional services sector. It highlights the growing adoption of GenAI tools across industries like legal, tax, accounting, and government, and discusses the challenges and opportunities these technologies present. The report also examines professionals' perceptions of GenAI and the need for strategic integration to maximize its value.
Read more